Biography
I am a second-year Ph.D. Student at University of California, Merced, advised by Ming-Hsuan Yang. Currently, I am also a research intern at Creative Vision team in Snap Inc., where I work with Aliaksandr Siarohin and Sergey Tulyakov. My recent research interests are controllable video synthesis and creation. Previously, I obtained my M.S. and B.S. degrees from National Taiwan University, where I worked with Shao-Yi Chien. If you would like to learn more about me, here is my [CV] (updated in June 2024) or reach out to me at tsaishienchen [at] gmail.com!
I am honored to receive Graduate Student Opportunity Program Fellowship.
Selected Publications
Panda-70M: Captioning 70M Videos with Multiple Cross-Modality Teachers
We introduce Panda-70M, a large-scale video dataset with high-quality automatic caption annotations.
Computer Vision and Pattern Recognition (CVPR), 2024
Snap Video: Scaled Spatiotemporal Transformers for Text-to-Video Synthesis
We introduce Snap Video, a transformer based Text-to-Video model, allowing us to efficiently train a T2V model with billions of parameters for the first time.
Computer Vision and Pattern Recognition (CVPR), 2024
[Highlight, acceptance rate: 2.8%]
[Highlight, acceptance rate: 2.8%]
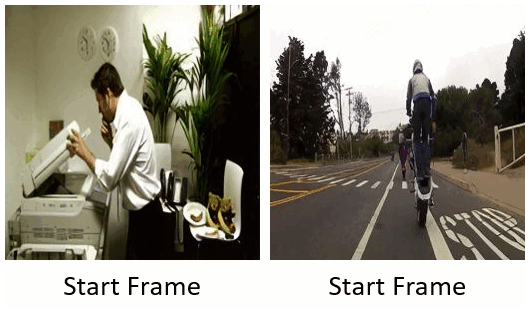
Motion-Conditioned Diffusion Model for Controllable Video Synthesis
We introduce MCDiff, a conditional diffusion model that generates a video from a starting image frame and a set of strokes.
arXiv preprint, 2023
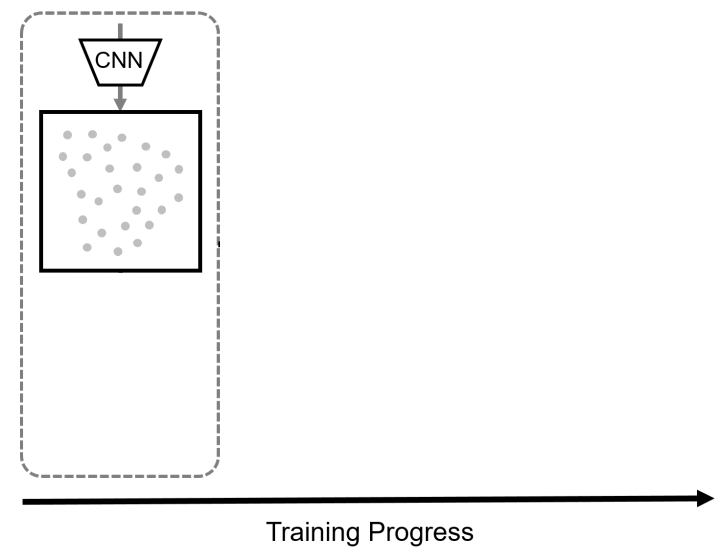
Incremental False Negative Detection for Contrastive Learning
We highlight the unfavorable effect from false negatives for self-supervised contrastive learning. To address the issue, we introduce IFND. Following the training process, when the embedding space becomes more semantically structural, IFND would incrementally detect more reliable false negatives and explicitly remove them during contrastive learning.
International Conference on Learning Representations (ICLR), 2022
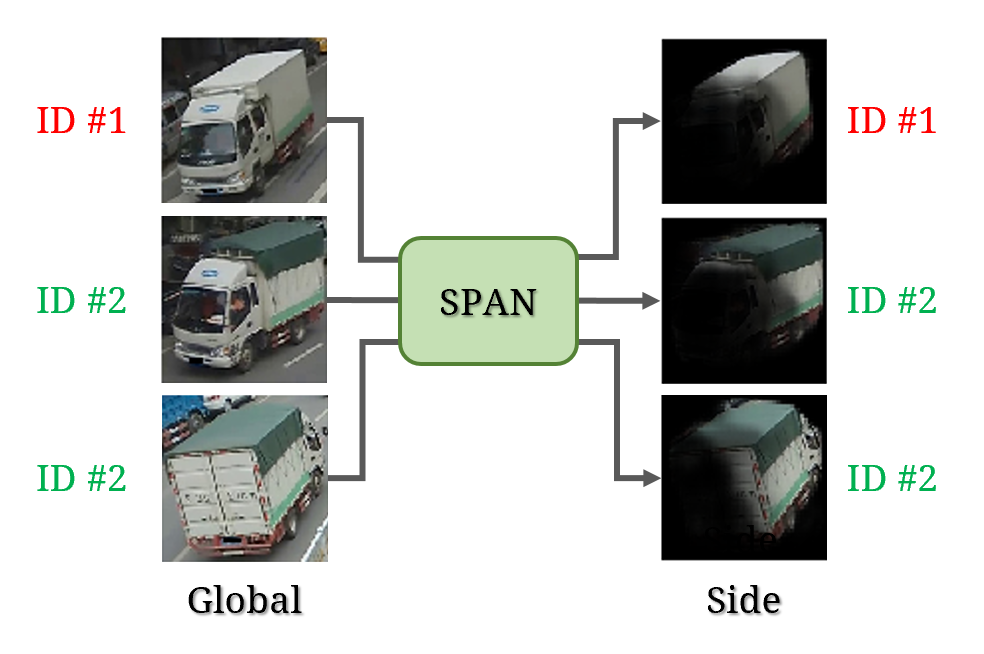
Orientation-aware Vehicle Re-identification with Semantics-guided Part Attention Network
In this paper, we propose SPAN to predict the spatial attention map for each vehicle view given only image-level label for training. We also introduce a distance metric emphasizing on the difference in co-occurrence vehicle views.
European Conference on Computer Vision (ECCV), 2020
[Oral, acceptance rate: 2.1%]
[Oral, acceptance rate: 2.1%]
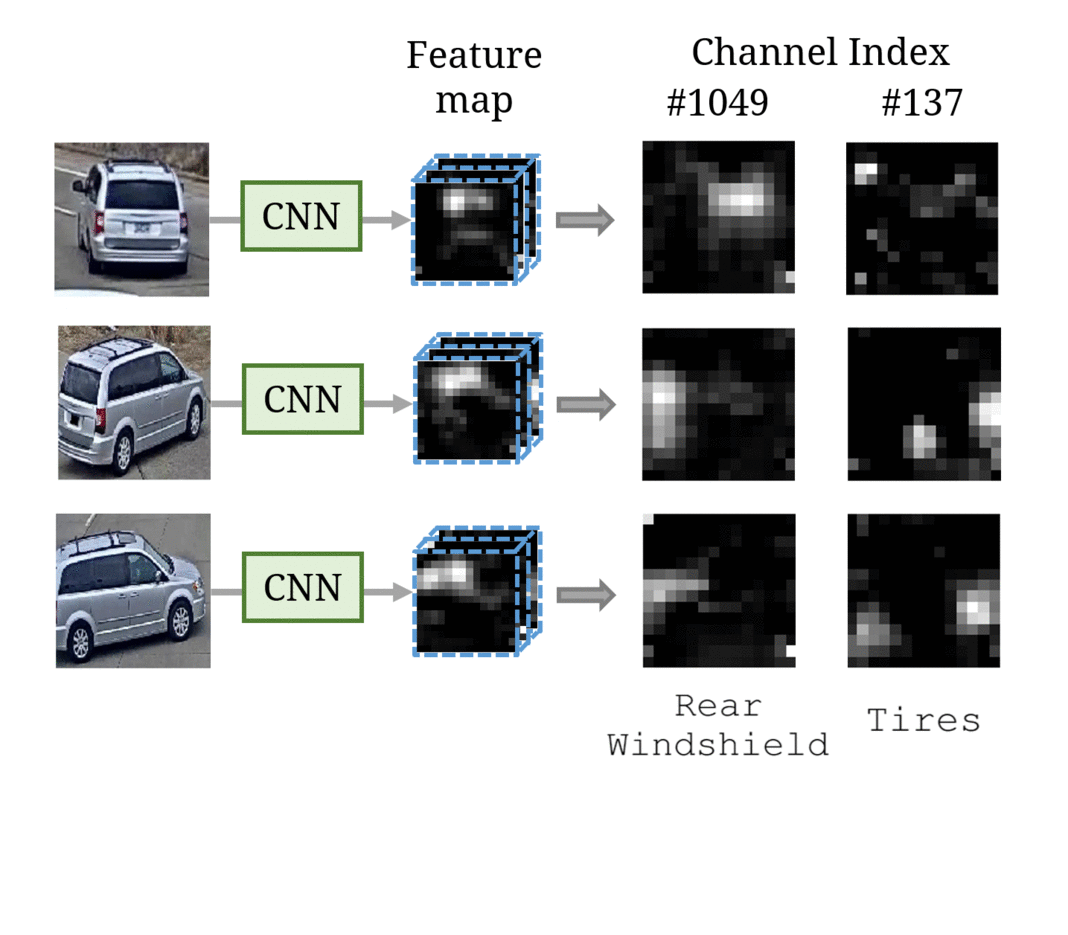
Viewpoint-Aware Channel-Wise Attentive Network for Vehicle Re-Identification
We propose VCAM to enable our framework channel-wisely reweighing the importance of each feature map according to the viewpoint of input vehicle image. By the aid of VCAM, we obtain promising results on 2020 AI City Challenge. We also explore the interpretability of how VCAM actually improves the performance.
Computer Vision and Pattern Recognition (CVPR) Workshops, 2020
Top